whitepaper
Generative AI for better risk management in Banks / Financial Services
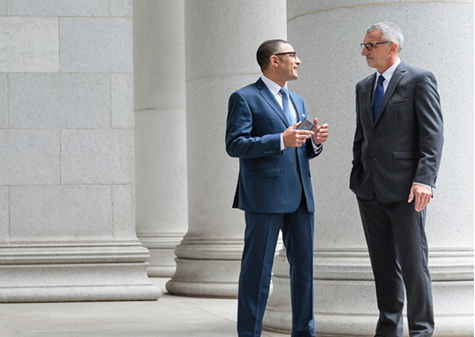
CONTRIBUTORS
This paper reflects on the findings of a project to explore the use of intelligent automation for controls testing for a major global bank.
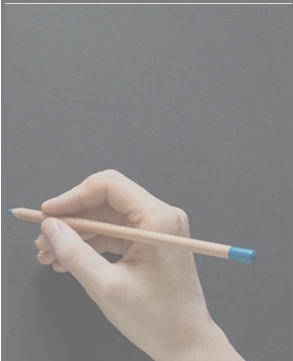
FOREWORD
There is a great interest in organizations in the promise of intelligent automation which combines Robotic Process Automation, Analytics , AI technologies along with Human-in-the loop and Process mining Techniques to get a better and seamless automation[1] to unlock increased efficiency and improved performance. This promise is fast becoming reality with growing adoption of intelligent automation by organizations.
INTRODUCTION
A test of controls is an audit procedure, to verify the effectiveness of key controls which mitigate against material risks. A test of controls may include verification of a sample of documents related to transactions that occurred throughout the year [2]. Doing so provides evidence that the system of controls has operated in a reliable manner throughout the reporting period.
Operational Risk (OR) is the potential for loss arising from the failure of people, process and/ or technology and may originate within an organization or from the impact of external events. Organizations perform controls sample testing to audit the effectiveness of controls in place for various processes and sub processes.
Operational risks have become far more complex, increasing the potential for failed processes that cause customer dissatisfaction. Effectively managing risk in the current uncertain and volatile environment will demand new capabilities and a rethinking of how risk management operates. Organizations are being forced to become more proactive when it comes to managing operational risk. By operational risk standards, 2019 was a modest year. Its $17.4 billion in losses look almost cursory next to the behemoth amounts of the recent past: $42.1 billion in 2018, $28.2 billion in 2017 and the astounding $56.9 billion of 2016 [3]
With the emergence of new technologies, transactions can be processed at much greater scale and speed than before leading to new types of potential failures and a need for appropriate governance. Weak controls, if undetected in STP (Straight Through Process), will lead to significant financial, legal and reputational loss for an organization. It is imperative that, given this scenario, organizations improve the effectiveness and timeliness of their risk identification methods.
Proactive risk management can be achieved by employing intelligent automation to automate repetitive manual activities involved in controls testing. Automation can reduce error rates from manual processes, can address human variability in testing and make risk identification more consistent.
CONTROLS SAMPLE TESTING
As part of transaction execution, various data files are produced. These data files could be swift messages, screenshots gathered in excel, PDF files etc. Such source data files for a set of sample transactions are gathered and then subjected to controls sample testing. Controls sample testing involves various checks to identify any exceptions in the process or a sub process to determine whether a control was effective or ineffective. These checks validate various data attributes from one source file to another or multiple source files and help in determining the conformance of transaction execution. The results are then fed into the organisation’s operational risk governance for review, assessment of the operational residual risk and, where necessary, remediation of the process controls.
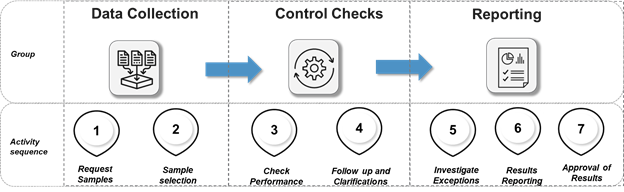
Diagram illustrates life cycle journey of control sample testing and sequence of activities executed manually
1.Collect data from various sources for completed transactions as per defined periodicity
2.Select samples
3.Perform checks to prove effectiveness of a control in transaction processing
4.Liaise with operational teams to discuss and clarify any exceptions
5.Investigate the exceptions in detail and assess residual risks
6.Report exceptions to risk governance committees within organization
7.Approvals to strengthen failing controls with mitigation
VISON FOR AUTOMATION
Most organizations perform controls sample testing manually to identify operational risk. Organizations have adhered to a culture of reactive damage control, but this is no longer sustainable considering the risks involved.
Using manual testing, a fixed number of samples are taken from the total population of a process or sub process and are subjected to several checks as part of controls testing. Exceptions arising from the validation are reported to evaluate the effectiveness of controls and to identify areas for process improvement. Many such control tests are in place in an organisation.
Based on the current process, a two phase approach is suggested whereby initially manual sample testing is replaced by automated control effectiveness tests and then, once comfortable with the technology, replace manual controls with automated controls and switch control effectiveness testing to an assessment of the working of the technology.
Vision |
Nature |
Method |
Result |
---|---|---|---|
Short term |
Reactive and automated |
Application of intelligent automation to control sample testing |
Transaction process not impacted. |
Long term |
Proactive and automated |
Change controls from being detective to preventive |
Transaction process re-imagined and transformed. /td> |
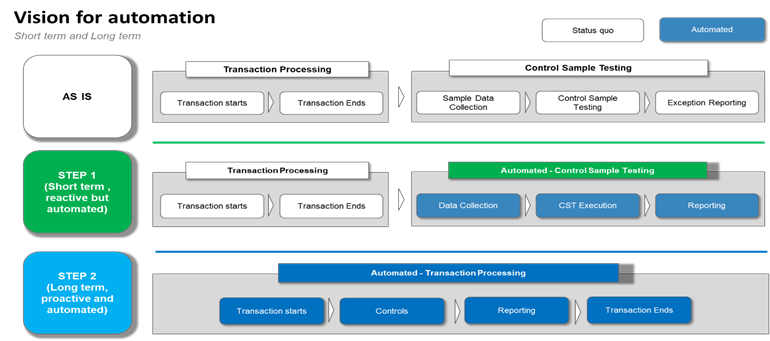
INTELLIGENT AUTOMATION
Testing and assessment of the effectiveness of controls continues to be a key focus area for organisations. Organisations make use of three lines of defence to manage internal controls. Control testing is typically executed by the First Line of defence. Despite the three lines of defence, organisations are faced with many challenges in identifying where operational loss may arise.
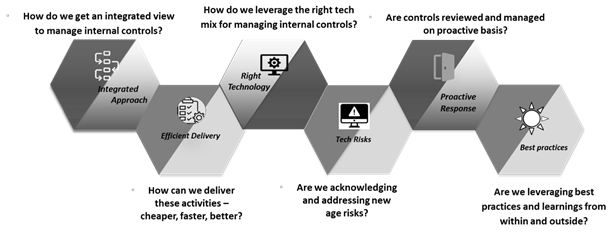
Organisations can leverage intelligent automation and its components for control testing. Gartner [1] has outlined the constituents of an intelligent automation solution. Such a solution would have one or more of the below:
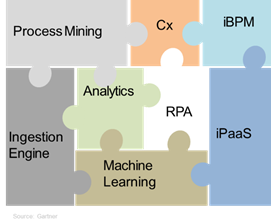
Combining RPA, Analytics and AI technologies along with Human-in-the loop and Process mining Techniques to enable a consistent and seamless E2E Automation
Manual or automated process mining to facilitate business understanding, process mapping and to outline the control testing process and automation needs. With a better understanding of the processes and common points of failure, users can redesign their processes.
Control testing automation at a minimum requires RPA, orchestration of workflow (automated and manual for managing solution exceptions) and AI enabled OCR.
Cx to add value in terms of interaction with operational users to enable smooth management of exceptions
Analytics and visualization to provide summarized- and detailed views of results along with various segmentation of identified exceptions.
APPLYING INTELLIGENT AUTOMATION FOR CONTROLS SAMPLE TESTING
Achieving short term vision – Control Testing Automation
Control testing automation requires a structured approach for selection of tests, reviewing them for return on investment / stated objectives and deliverability.
Selecting control testing for automation project
Identifying the readiness and value of control tests for automation requires an assessment of various selection criterion. A scoring method can be used to quantify the feasibility of automation. The table below proposes an assessment approach.
Sr. No. |
Criteria |
Description |
Example |
Prioritisation |
---|---|---|---|---|
1 |
Stability of control effectiveness. |
Assess the number of exceptions and/ or the residual risk rating over 6- or 12-months period. |
If there have been no material exceptions which warranted a change in residual risk assessment, the process / sub process can be considered stable. Otherwise based on the nature and frequency of exceptions, tests should be rated as lightly, moderately or highly unstable. |
Prioritize tests where the control is unstable. Automation of the control testing will allow more extensive sampling (greater confidence in results), improved timeliness (faster awareness of issues) and better understanding of the source of control ineffectiveness (better analytics). |
2 |
Whether a control test uses digital data. |
Verify if the check uses digital data from transaction processing systems to perform the control test. |
If the control test requires the verification of a signature stored in a database, the stored signatures would be considered digital data. |
Understand the data being used for the control testing. Group control tests by data type (which informs the type of automation capability required) and prioritize development where there are clusters of tests using similar data types. |
3 |
Whether a control test is objective. |
Verify if the check is objective, with limited interpretation of results required and a set of binary/ finite outcomes |
If a control test requires verification to ensure that a checklist has been completed on a new client instruction, the check is binary and objective as either a checklist is present or not, |
Control tests which are objective in nature, without subjective interpretation, are simpler to develop than those that require a level of interpretation. Objective tests can be programmed using simply logic expressions without any need for AI. |
4 |
The degree to which a control test is subjective. |
Verify the degree to which a test requires subjective interpretation of the results of a control test |
If a control test requires verification of the authenticity of a signature on a client instruction against a sample signature, there is a degree of subjectivity about interpreting the result of that check. The permutations of possible signatures are infinite. |
Subjective tests require some level of interpretation and, to be automated, necessitate the application of artificial intelligence. The more complex the test, the more complex the AI required. Cluster tests based on complexity and consider prioritizing simpler tests first. |
5 |
What type of documents are checked in a control test |
Verify whether a control check requires a document-to-document check. If yes, detail what kind of documents are compared. |
Email to screenshot |
Cluster and prioritize tests based on the types of document being reviewed. Clustering enables efficient and focused roll-out of capability. |
6 |
Assess the extent of non-standard data files used in a control test. |
Verify whether the data involved in the control test is standard or has multiple possible forms/ is non-standard. |
Checking a free-format email for key words. |
Non-standard data may need more time and effort to automate so prioritize those with a higher degree of standard data. |
Review of Objectives and ROI
Organizations can automate unstable process-related control testing, thereby increasing the coverage of the test and frequency of such test execution.
Organizations can as well automate the stable control checks to improve efficiency and allow the team to focus on those controls that are failing as well as minimizing the risk of initial failures with automation.
The type of check is a major factor as data extraction from source documents influences the automation accuracy. Data extraction from source files could be as simple as extracting data from an Excel spreadsheet or could be as complex as inferencing various images, segmenting them and then extracting data from the images for a specific product.
Depending on the complexity of the extraction process, the trade-off between accuracy and process change management needs to be managed. Similarly, the trade-off between an automated solution and return on investment (ROI) needs to be managed. Control testing automation, by default, enables flexible sample selection and improves coverage, thereby allowing the operational teams to increase or decrease the samples used based on operational risk assessment.
Automate – Life cycle journey of a control test
The life cycle of a control test is evaluated in terms of indicative turnaround time, % automation feasibility, pain points with manual execution and potential benefits from automating the activity
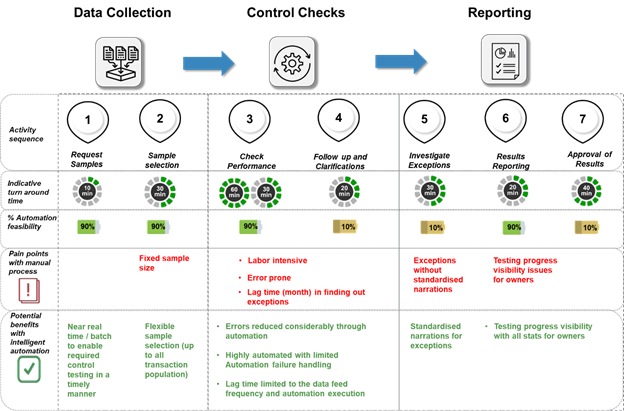
Intelligent automation can automate many of these activities with a solution for each control sample test, from collecting data to reporting the exceptions. The table below depicts the intelligent automation component (as outlined by Gartner) and thus the objectives set for a typical automation project.
Activity Sequence |
Activity Name |
Intelligent Automation Component |
Automation objectives |
---|---|---|---|
1 |
Request sample |
RPA |
Samples collected in a scheduled manner. |
2 |
Sample selection |
RPA, Ingestion Engine, Machine Learning and iBPM |
Number of samples collected can be expanded up to complete transaction population. |
3 |
Check performance |
RPA, Ingestion Engine, Machine Learning and iBPM |
Objective checks automated. |
4,5 |
Investigate exceptions |
iBPM and Cx |
Standardised narrations for exceptions resulting from automation enables better and faster investigation and analysis |
6 |
Results Reporting |
Analytics and Cx |
Automation dashboards enable better visibility of execution, exceptions and allow users to have detailed drill down of data and analysis |
Factors for automation sustainability
Creating an environment that enhances trust with respect to deployed automation is critical.
There are certain new factors that needs addressing by experts such as, solution fall outs / exceptions arising out of automation, new kinds of data as part of checks which are not managed by automation. Addressing these factors by design facilitates the sustainability of automated solutions and enables operational users to manage new situations.
1.Solution fallouts could be due to quality issues with input data. In such cases, the expert may need to perform a manual control test. If solution fallout is due to exceptions from automation software, then it needs to be fixed as part of the automation in-life support.
2.If there are new kinds of data being seen (for example, changes to control parameters), then the change management process needs to be invoked to consider changes to the checks being performed. Ideally automation should be built flexibly, keeping in mind the likely adaptions required over time
Achieving long term vision – proactive controls
Organizations, once matured with control testing automation, can re-imagine the transaction processes.
One such enhancement could be the potential re-use of automation built for controls testing for setting proactive controls during transaction processing. Such enhancement would be fruitful for transactions that are executed in batch mode. Though this is seen as a long-term vision, some of the capabilities built by automation can be evaluated for early adoption into transaction processing, such as signature matching, know-your-client list matching.
There will be processes that severely impact user experience, even with slightest delay. Such processes would need to evolve over time with strengthened STP (straight through processing) based on the experiences and learning from automated control testing. Operations team can leverage the reactive controls testing automation to flexibly select samples, schedule executions and exercise much higher control than today.
Use case – Mark-to-market testing in Financial Markets
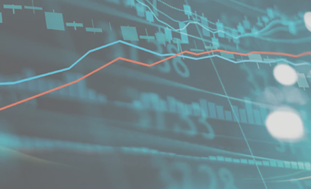
Operational oversight in the Financial Markets context assumes critical importance due to its financial, regulatory and cascading impact and repercussions on business and reputation.
In the case of mark-to- market (MTM), the mark used is critical for accurate evaluation of the profit and loss accounting of traders’ positions, assessment of client credit exposure and the business’ margining requirements. The mark must be assessed against the market benchmarks for accuracy.
A manual control test validates the marks detailed in a working file against the marks used in the client valuation statements (manual or system generated).
Achieving short term vision – control test automation
In the short term, Intelligent Automation enables automated collection of source files (working file and the client valuation statements). Samples are then selected for control testing using business rules. An AI enabled OCR solution extracts data attributes like the deal number, MTM % and notional value and currency. A rules engine can compare these extracted details from different source files and perform objective and subjective checks.
The resulting discrepancies are reported as exceptions in a summary report for all deals and a detailed report per deal highlighting the specific data attribute exceptions. In this way, the automated solution provides an effective and efficient means for testing the accuracy of the manual client valuation statements.
Proactive controls
Intelligent automation, in the long term, enables the automation of manual controls, from trade confirmation to trade settlement, on a real time basis. For this to happen, organizations need a degree of comfort that the intelligent automation they put in place is operating to desired standards.
SUMMARY OF PAIN POINTS IN MANUAL EXECUTION AND BENEFITS FROM AUTOMATION
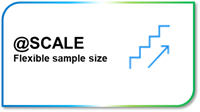
pain points
A fixed number of samples of transactions along with source data is selected and subjected to controls testing. This does not provide true reflection of how the controls operated at specified period.
Automation Benefit
Automation allows flexible selection of samples and adjust the number of samples based on risk assessment and outcome of controls testing.
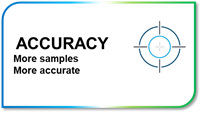
pain points
Controls test are manually executed for the selected samples of transactions. Accuracy of outcomes reported is always questionable.
Automation Benefit
Accuracy of outcomes reported is consistent, residual risk assessment improves with many samples considered for controls testing.
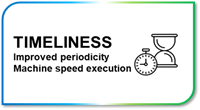
pain points
Operational teams perform a tightly scheduled manual assessment and reporting of exceptions. This is generally done with lag time of month or two post transactions being executed.
Automation Benefit
Automation enables timeliness with a higher frequency of samples on real / near real time basis of transactions being executed.
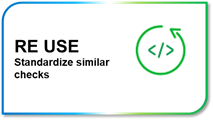
pain points
Operations of various lines of businesses within the same organization could be performing similar checks manually.
Automation Benefit
Similar checks are automated consistently across organization with automation and with high re-use of automation solutions.
CONCLUSION
Covid-19 situation has enabled organizations to achieve transformations which was deemed unattainable earlier. This situation has provided opportunity to organizations to re-imagine back office operations and increase the priority for automation. Organizations can taper the disconnect between words and actions in challenging the status quo, create a more resilient, robust operations by encouraging a new phase of automation along with sustainability.
Automation so far has been seen as FTE (Full Time Equivalent) reduction measure, but the qualitative improvements delivered by automation such as higher speed of execution, improving accuracy of results, improving coverage of activities are key levers for operational teams to bring in efficiencies and support organizations for non-linear growth with the same number of FTEs.
Manual operational risk auditing processes are fragmented, siloed, ineffective, and unsustainable with emerging risks from digital technologies. A comprehensive digital operation focused on delivering business outcomes can automate every aspect of the value chain of operational risk management. A combination of advanced technologies blended with key focus on delivering business outcomes through technology partners and valued startups can transform organizations.
REFERENCES
1.Gartner – hyper automation 2020
2.Test of controls ,Steven Bragg, 2019 - https://www.accountingtools.com/articles/what-are-tests-of-controls.html
3.https://www.risk.net/comment/7272191/top-10-operational-risk-losses-of-2019